- Home
- NEWS
- News
- Reo Furuhata , Minglu Zhao(Takizawa Lab MC1), Mulya Agung(Takizawa Lab Researcher), Prof. Ryusuke Egawa(Tokyo Denki University ) and Prof. Hiroyuki Takizawa won the Best Paper Award at International Workshop on Large-scale HPC Application Modernization
Reo Furuhata , Minglu Zhao(Takizawa Lab MC1), Mulya Agung(Takizawa Lab Researcher), Prof. Ryusuke Egawa(Tokyo Denki University ) and Prof. Hiroyuki Takizawa won the Best Paper Award at International Workshop on Large-scale HPC Application Modernization
Reo Furuhata , Minglu Zhao(Takizawa Lab MC1), Mulya Agung(Takizawa Lab Researcher), Prof. Ryusuke Egawa(Tokyo Denki University) and Prof. Hiroyuki Takizawa won the Best Paper Award at International Workshop on Large-scale HPC Application Modernization
by the following paper:
Reo Furuhata, Minglu Zhao, Mulya Agung, Ryusuke Egawa and Hiroyuki Takizawa
Improving the Accuracy in SpMV Implementation Selection with Machine Learning
There are various ways of implementing a sparse matrix vector multiplication (SpMV). An SpMV implementation has empirically been selected for each matrix. In this paper, we proposed an approach for selecting an appropriate SpMV implementation using machine learning. The previous approach focuses only on predicting the best implementation for each matrix and does not consider the performance differences among candidate implementations. In contrast with the previous approach, the proposed machine learning approach considers the performance differences at the best SpMV implementation selection problem.
The proposed approach can prevent a machine learning model from selecting significantly low-performance implementations, and thereby improve the expected performance in comparison with the previous approach.
Takizawa Laboratory
https://www.sc.cc.tohoku.ac.jp/en/
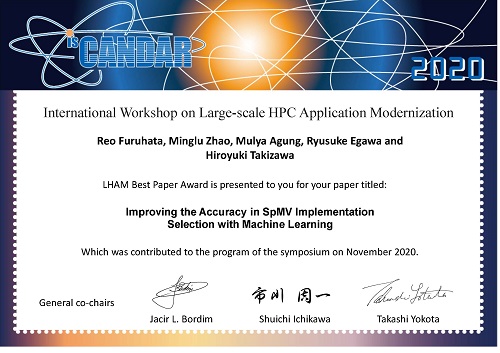